The adoption of Artificial Intelligence (AI) is accelerating as organizations strive to achieve technological advancement to bolster their business objectives. As organizations embark on these initiatives, many of them are implementing AI projects as if it was an analytic solution or even a transactional system. This is the AI Minefield. There are many nuances to AI implementations that are considerably different from the traditional software implementation projects that have been conducted over the last 30 years.
Understanding the AI Approach
AI models are rarely fully executable without human intervention. Keep in mind AI is a combination of pattern matching leveraging advanced mathematics and statistical equations. AI also works in tandem with much larger systems. With all these systems and data, focus must be placed on human interaction and monitoring.
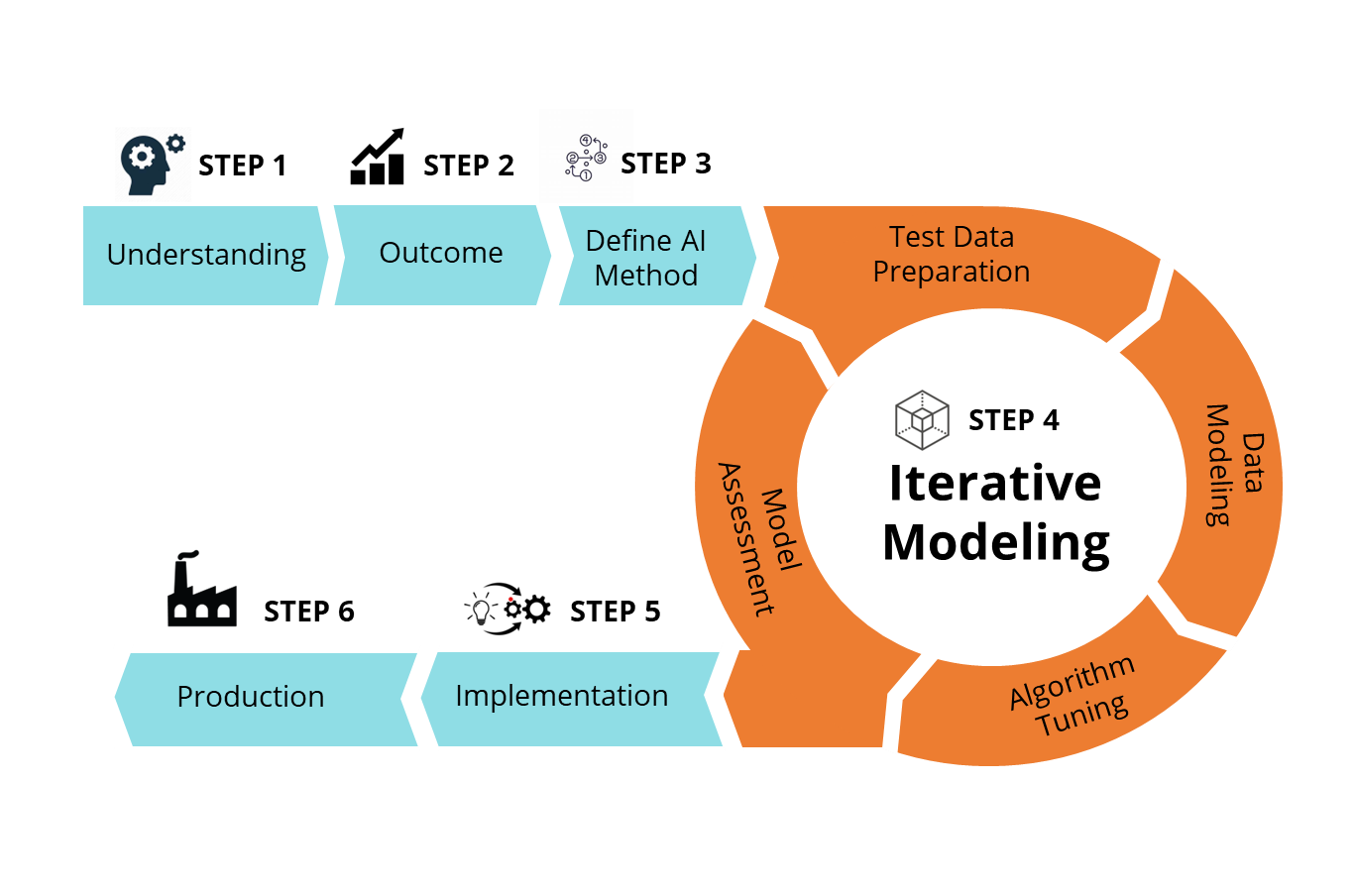
Identifying AI Minefields Early On
The AI mines are scattered throughout an AI initiative. It’s important to know what those mines appear in the beginning before you are even fully invested. Even Gartner recommends three different phases of an AI project which are essentially: Strategy, Testing, and Implementation. Even though these phases are linked together, it's recommended that each phase is treated as a phase gate. This allows a decision point at each phase to determine whether to continue or pivot.
Common Land Mines in AI Projects:
Lack of Clear Objectives: It is critical to strategize on the possible use cases for AI within your organization, then prioritize one focus area to explore further. It’s important through a strategy workshop to identify more than one focus area in the event the top choice is not feasible due to data gaps or complexity.
Lack of Stakeholder Buy-in: If key stakeholders, such as executives, end-users, or decision-makers, are not aligned or supportive of the AI project, it may struggle to gain traction and eventually fail.
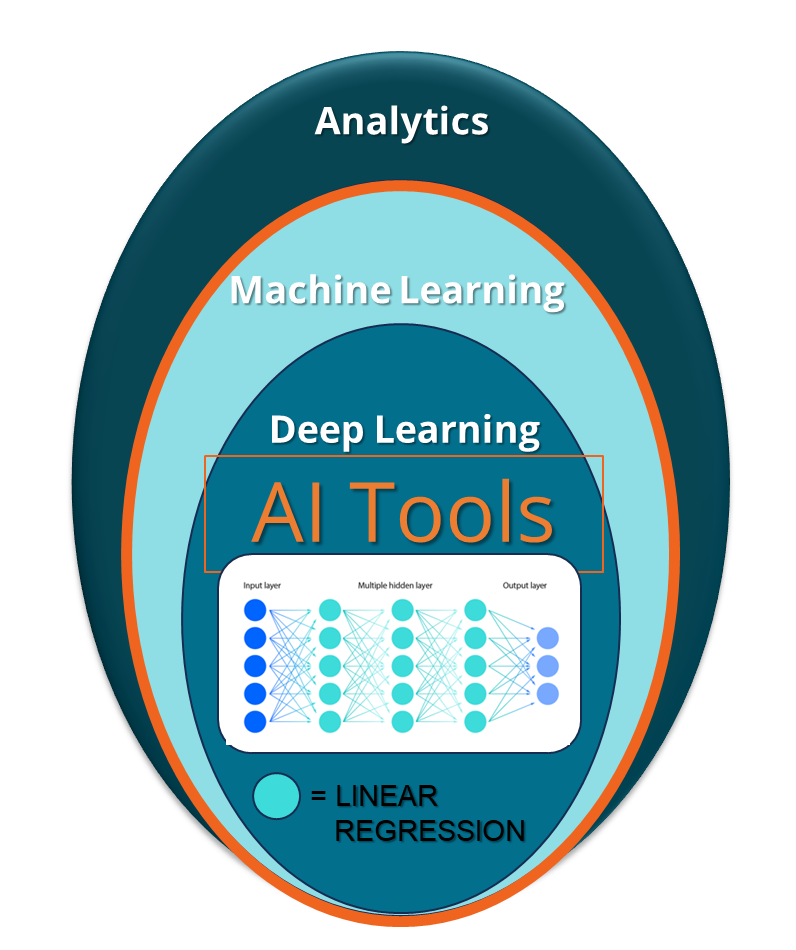
Overly Ambitious Goals: Attempting to tackle too complex problems with limited resources or technology can lead to failure. It's important to set realistic goals and expectations. This also goes back to a limited scope of only a few focus areas.
Poor Model Selection: Choosing the wrong AI method, algorithm or model architecture for the problem at hand can result in suboptimal performance or failure to achieve the desired outcomes.
Ineffective Implementation: Even if the AI model performs well in testing, if it's not effectively integrated into existing systems or workflows, it may fail to deliver value in real-world applications.
Poor Data Quality: AI models heavily rely on data and if the data used for training is of poor quality, incomplete, or biased, it can lead to inaccurate or unreliable results.
Data Drift: If the new data entering the system is slowly changing or the context of that data is changing then this can cause issues once the solution is in production. The key to mitigating this issue is by monitoring the output against expectations.
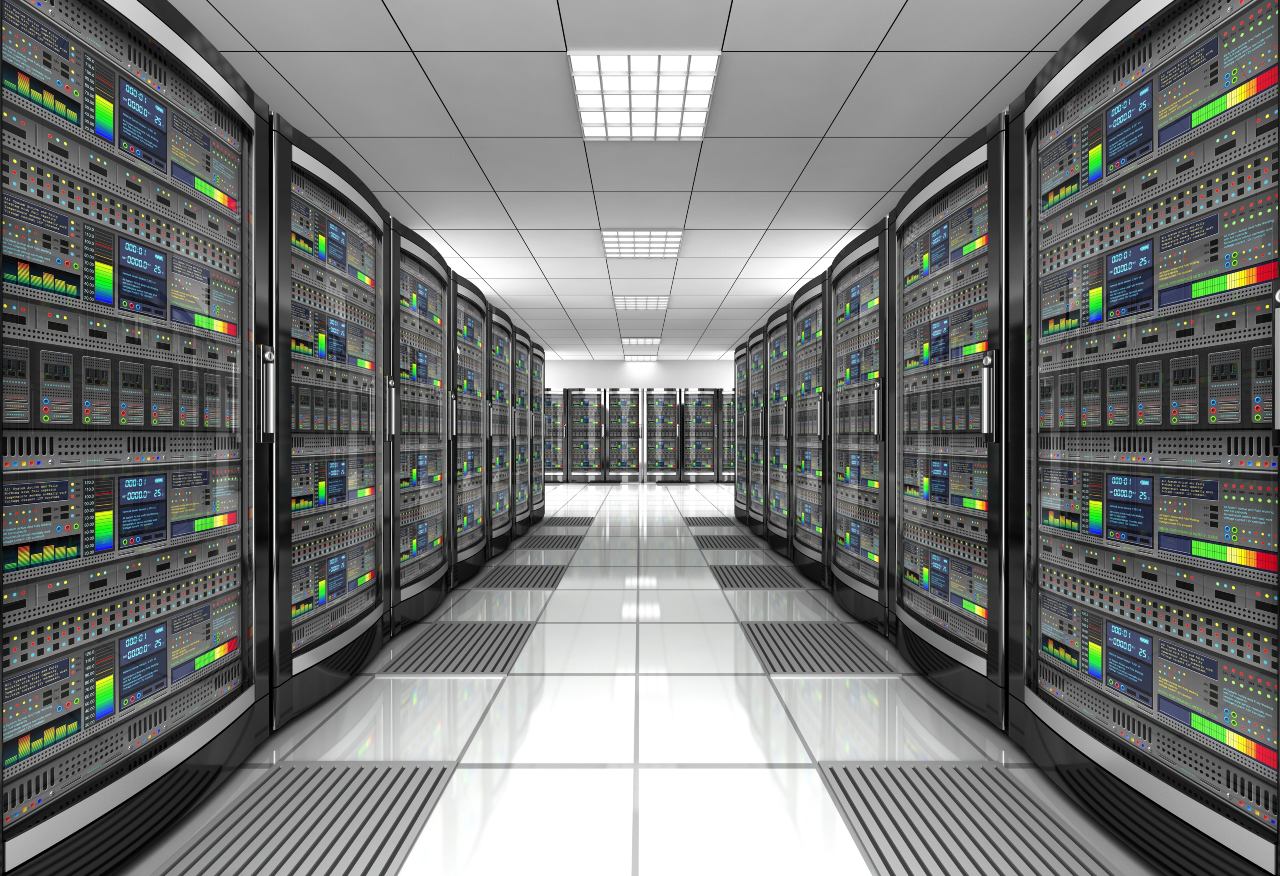
Insufficient Data: In some cases, there might not be enough relevant data available to train a robust AI model, leading to underperformance or failure.
Ethical and Legal Issues: AI projects may face challenges related to privacy, security, bias, fairness, and compliance with regulations, which can lead to failure if not adequately addressed. This is an area where personal identity information (PII)and other privacy compliance regulations can impact the success of the solution.
Lack of Expertise: AI projects require interdisciplinary expertise, including data science, machine learning, domain knowledge, and engineering. If the team lacks the necessary skills or experience, it can lead to failure.
Charting a Course for AI Success
Overall, successful AI projects require careful planning, sufficient resources, interdisciplinary collaboration, and ongoing monitoring and iteration to address challenges as they arise. Developing a plan to address these potential obstacles before starting your AI project gives you (and your model) the best chance of success.
Having implemented over 50 AI projects what has worked best is the phase gate approach which again is: Strategy, Model Iterations/Testing, Implementation/Monitoring. This gives the organization control with clear points from which to pivot. Not every AI initiative is successful, so the key is to identify that early and adjust so that you can impact your organization.
Written by Kyle Johnstone
Kyle Johnstone has over 30 years in data and analytics and serves as Wimmer Solution Practice Director. Kyle has implemented nearly 50 AI projects in the last 10 years. Kyle has served with IBM, Arthur Andersen, E&Y, SAP, and Hitachi Solutions over his career.